Since the emergence of Chat GPT, artificial intelligence has drawn a lot of attention. The discussion highlighted important topics, such as ethical and security concerns, that still continue to shape AI-powered tools of today.
However, the rise in attention also led to a flood of misconceptions. While some of them are harmless, most are misleading and create a false impression of AI.
Altamira’s Chief Technology Officer, Alex Budnik, shared his insights and dispelled common myths about AI.
Myth #1: All AI systems are “black boxes”, and nobody understands how they think
How do you know that Machine learning (ML) and deep learning models output correct information if you don’t know how they arrived at their conclusions? Unlike traditional software that has explicit rules, ML bases conclusions on the trained data. Models consume a lot of information that is processed by complex internal structures, making it difficult to trace the decision-making process.
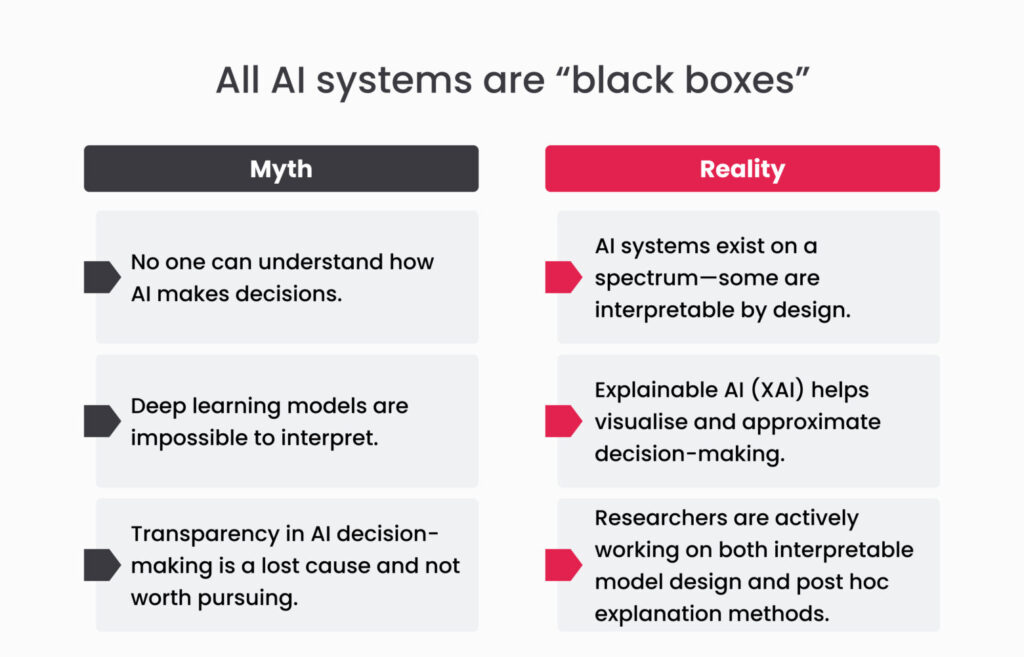
The problem becomes even more obvious in deep learning models since they’re based on neural networks that mimic the human brain.
The “black box” issue raises legitimate concerns in critical applications. For example, a recent review about AI in healthcare has found that 94% of 516 ML studies have failed to pass even the first stage of clinical validation tests.
In finance, Laura Blattner, an assistant professor of finance at Stanford Graduate School of Business, has explained that: “The power of technology is its ability to reflect the complexity in the world. But the lack of understanding raises practical, legal, and ethical questions.”
The reality
Saying that “all AI systems are black boxes” is an oversimplification. It is true that complex deep learning models can be challenging to interpret, but many other AI approaches offer better transparency.
A recently emerged field of explainable AI (XAI) focuses on making AI decisions more transparent, even though most XAI techniques operate post hoc and can only approximate the model’s decision-making process.
Many researchers are actively working to make AI more transparent and interpretable. Some of the efforts include:
Developing inherently interpretable models
Creating tools for visualising and explaining model decisions
Establishing frameworks for AI governance with a focus on transparency
Concerns about bias, opaque reasoning and regulatory gaps still exist, but these efforts are only the beginning in building trustworthy AI systems.
Myth #2: AI is approaching human intelligence
This belief was born from the popular media and fuelled by tech industry narratives. Stories of AI outperforming humans in chess, composing music, or generating realistic text only deepened the misconception that machines are quickly catching up.
Bold predictions don’t help with the issue either: some forecast that artificial general intelligence (AGI), a system that can perform any intellectual task, will be created within years.
While the narrative might sound compelling, it’s still far from the truth.
The reality
Nowadays, AI excels at narrow tasks, such as:
Identifying patterns in large datasets
Generating plausible-sounding text
Making predictions based on statistical correlations
However, these capabilities shouldn’t be mistaken for human intelligence; the output simply reflects patterns learned from data. AI doesn’t understand what the outputs mean.
The key AI limitations are:
Lack of generalisation: Models trained to detect tumours in medical scans can’t shift to diagnosing pneumonia or interpreting the emotional tone of the conversation
Absence of common sense: AI systems fail at reasoning through routine scenarios, making contradictory or nonsensical claims
No real understanding: Language models can generate sentences by predicting which word should go next, but they don’t grasp the meaning of the said words
The human brain is an example of flexible, self-aware, and context-sensitive intelligence. It can learn from a few examples, adapt to changes, and reason intuitively. Humans have emotions, memory, perception, and judgment.
In contrast, AI is narrow, data-hungry and rigid. The most advanced models still require massive computational resources to achieve the same results a child could — like understanding a joke or adapting to a social situation.
The myth that AI will soon surpass humans is partially a consequence of its design. Modern AI is built to mimic human language and decision-making patterns. It’s easy to mistake a chatbot’s crafted heartfelt message for depth. In reality, these systems are just manipulated symbols without any understanding of their meaning.
Myth #3: AI will replace all human jobs
AI’s impact on employment is one of the most pressing concerns. According to the World Economic Forum, AI and automation will displace 85 million jobs by the end of 2025, and 97 million new jobs might emerge.
This highlights the dual nature of AI’s impact: some jobs will disappear, but new positions will replace them.
Certain types of work face a higher risk of displacement by AI automation. For example:
Routine cognitive tasks, such as data processing, basic analysis, and rule-based decision-making
Structured physical labour involving repetitive manual tasks in controlled environments
Basic customer service functions, such as standard inquiries and information provision
The reality
While AI substitutes some jobs, it also creates new ones in fields like data analysis and programming. A surge of AI adoption across industries creates demand for AI specialists, data scientists, AI ethicists, and jobs requiring human skills, such as creativity, empathy, or complex problem-solving.
AI’s employment impact varies across industries. For example, healthcare is embracing AI for advanced diagnostics, personalised treatment, and predictive analytics, transforming healthcare jobs. In transportation, AI enables autonomous vehicles and optimises traffic management and logistics. Retail and e-commerce benefit from AI-powered customer support, personalised recommendations, and automated inventory management.
However, to help workers adapt to AI-driven changes in the workforce, they need education and training.
Since routine tasks become automated, jobs will start to highlight unique human capabilities, such as creativity, critical thinking, emotional intelligence, and complex problem-solving.
The transition will require significant investment in reskilling and upskilling programmes and education reforms to prepare students for an AI-driven workplace.
See also: The future is now: AI deep dive
Myth #4: The more training data AI has, the smarter it will become
It’s easy to see where the “more data equals better intelligence” mindset comes from. The logic is based on high-profile projects that train AI models on massive datasets.
The myth only gained momentum from real-world examples, like Openai’s GPT-4. The results seemingly confirm the idea that more data leads to smarter AI.
The reality
It’s true that more data can improve performance, especially in the early stages of model training. However, beyond a certain threshold, adding more data will give only marginal gains. In some cases, excessive data overwhelmed or confused the model. This issue is even more pronounced with low-quality, redundant, or inconsistent data.
Bigger datasets also require more computational power, longer training, and higher costs, a substantial price to pay for a chance to improve the AI model.
Additionally, these datasets don’t help AI systems develop a deeper understanding of the world. Such systems only become better at identifying patterns and correlations, but they don’t gain common sense, context awareness, or conceptual reasoning. Language models are trained on billions of sentences but still fail basic logic puzzles or misunderstand cause-and-effect scenarios.
Building a smarter AI isn’t about using more data. It’s about different kind of learning that integrates reasoning and abstraction, challenges that can’t be solved by brute-force data ingestion.
Myth #5: AI, machine learning, and deep learning are interchangeable
The terms AI, ML, and deep learning are often used interchangeably in popular discussions. Articles, marketing materials and even technical blogs sometimes mix these terms or just refer to a system as “AI-powered”.
The fact that these terms refer to the same concept doesn’t help either. For people outside the field, “AI” might seem like a label applied to any algorithm.
The reality
All three terms refer to slightly different things.
Artificial intelligence refers to the science that enables computers and machines to simulate human learning, comprehension, and problem-solving. In simple terms, the goal of AI is to create intelligent machines.
This is an umbrella term that includes both machine learning and deep learning.
Machine learning is a subset of AI that enables systems to learn from data instead of being explicitly programmed. Once trained, ML algorithms can find patterns and make decisions with minimal human intervention.
ML is one of the strategies used to make AI.
Deep learning is a subset of ML that uses layers of neural networks to learn complex features from data, especially unstructured, automatically. DL is one of the techniques used for ML.
Why these myths matter
Misconceptions about artificial intelligence aren’t just academic—they shape how AI is built, evaluated, and used. When people believe that more data always makes models smarter or that AI is already approaching human-level intelligence, it sets distorted expectations.
These myths can lead to poor decisions, misplaced investments, and mistrust from users who were promised one thing and experienced something entirely different.
In healthcare, finance, and education, misunderstanding how these systems work—or what they actually are—can slow down adoption or result in superficial deployments that fail to deliver.
Clearing up these misunderstandings requires transparent communication and grounded expectations. Recognising the real strengths and limits of AI opens the opportunity for better integration.
See also: Implementing AI: Is it worth it? A step-by-step guide to AI implementation
Final thoughts
Artificial intelligence is a technology of the future. However, it’s not a “magic wand” that can do anything humans can. Actively combating misconceptions helps people stay more informed and make smarter choices about the use of AI.
How Altamira can help
Whether you are looking to add the latest AI tools to your business, validate if your business is ready for AI adoption, or improve your products and services with the power of AI, our approach guarantees to reduce your stress.
Zero headaches: Get a team with a proven track record of pushing the boundaries of what’s possible with AI
Tangible value in days: Clickable prototypes even before a contract starts to demonstrate our commitment
Always stay in the loop: Have daily or weekly meetings with transparent communication so that you always know the state of your project
Contact us and take your products to the next level with AI.