Table of Contents
Every business has that one crucial system that has been around forever. For almost all of the company’s life, it was there — stable and reliable.
As the years pass, technologies improve and demands grow, that old system starts to feel the passage of time: it becomes less stable, and quite often becomes a burden on the organisation.
Most of the legacy systems could be modernised, but why not make them future-proof? Modernising legacy systems with artificial intelligence breathes new life, turning a burden into a new force that pushes businesses to new heights.
Why should you modernise legacy systems?
Legacy systems are often the backbone of business. What used to be innovation ten, twenty, or even more years ago has become outdated by today’s standards. Think pagers in a smartphone era.
However, the main problem isn’t that these systems are outdated; it’s how they impact your business today. Growing costs and integration issues are just the tip of the iceberg we’re about to uncover.
See also: The future is now: AI deep dive
Challenges of legacy systems
Legacy systems were built to meet the demands of their time. As the technologies evolve, these systems struggle to keep up, causing compatibility, performance, and integration issues. At some point in time, they transform from useful and powerful tools into a burden, taking from the business more than they offer in return.
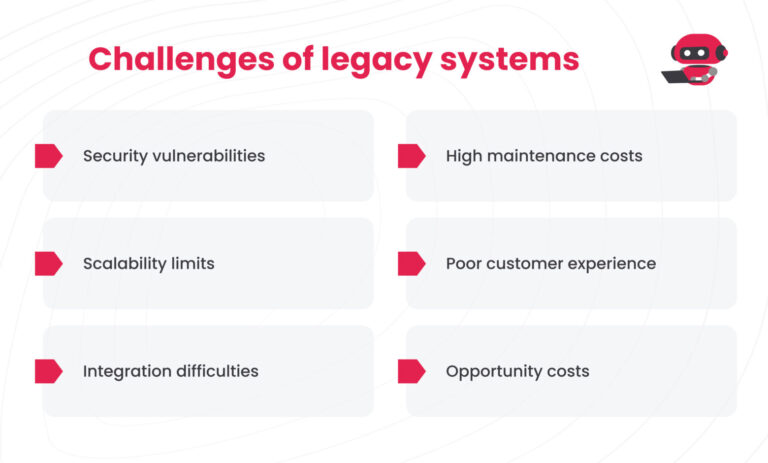
Security vulnerabilities
Nowadays, fewer and fewer systems receive updates and patches. While some might not like the perpetual “update now” buttons in today’s software, they exist for a reason.
Cyberattacks constantly evolve and change, finding new vulnerabilities almost every day. It’s a constant race between malicious actors and cybersecurity experts.
Usually, legacy systems aren’t supported by the original vendor, making their security subpar compared to newer ones.
Scalability limits
A lot of legacy systems encounter this issue. In the time of their development, engineers couldn’t even think about the modern scale of operations, which is why most of them fall behind on scaling.
With increased user demand and data volumes, such systems will underperform or break down entirely, forcing them to build workarounds. It does fix the system temporarily, but it also adds to the complexity of legacy systems.
Integration difficulties
Imagine working in the retail business somewhere in Europe, and your client starts speaking to you in Latin. This is a similar experience to how modern platforms connect to legacy systems.
What was once the newest connection protocol is now dust, and older architectures might be completely incompatible with modern APIs.
High maintenance costs
Legacy systems are great money sinks, especially if it wasn’t built in-house. Support contracts are just the tip of the iceberg — finding old hardware and employees that actually know how to support it are the real costly problems. It’s not easy to find relics from the past, let alone operate them.
Poor customer experience
Most legacy systems were built in a time when speed wasn’t the biggest priority — everything was much slower compared to modern times. Often you had to wait a minute or two before getting any results.
Nowadays, however, it’s a real issue that could lead to frustration and impact how customers see your business.
Opportunity costs
Money spent on a legacy system is the money you didn’t spend on improving your business. Just imagine how many growth opportunities you missed because you didn’t have a budget for them — and how many you’ll miss in the future for the same reason.
Benefits of modernising systems with AI
It’s clear that something needs to be done with legacy systems. But why modernise them specifically with AI? In short, AI will make such systems smarter, faster, and more adaptable. It’s not a temporary workaround; it’s an actual improvement.
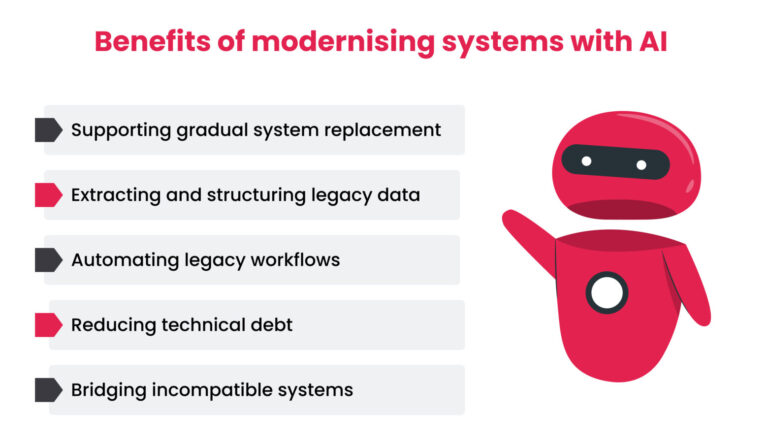
Supporting gradual system replacement
The most common solution to the issue with the legacy system is to take it down and replace it with a newer one. While effective, it’s still a risky approach; you never know how much you rely on that old system before you take it away.
AI can help extend legacy systems and support the introduction of new components.
Extracting and structuring legacy data
Legacy systems are a goldmine of valuable data. The only issue — it’s poorly structured. AI tools, more specifically natural language processing or data classification models, help extract, clean, and reformat old data so it can be migrated and used in modern apps.
Automating legacy workflows
Usually, processes in legacy systems are either manual or hard-coded. Manual processes are often cause issues, but hard-coded functions are harder to explain, let alone replicate.
AI can identify patterns in how the system works, replicate the logic behind hard-coded processes, and automate them. This way, manual tasks can be done without lifting a finger, and hard-coded functions become easy to update.
Reducing technical debt
Legacy systems, especially the ones that have a lot of patchwork on them, often burdened by technical debt. AI can analyse the code, identify which parts of the code are most used and which often fail.
Based on its findings, modernising legacy systems becomes easier, since you avoid the guessing game and know the functions of every part of the code.
Bridging incompatible systems
Remember that example with a client speaking Latin? Think of AI as a translator — it can normalise data formats, interpret inputs, and even bridge systems without direct integration
Suddenly, incompatible systems can talk to each other, making the data migration and transition to the newer systems more manageable.
See also: Implementing AI: Is it worth it? A step-by-step guide to AI implementation
Real-world examples of modernised legacy systems with AI
It’s common for modern businesses to have a legacy system. The struggle is real, but so are the solutions. AI doesn’t bring benefits just on paper; many companies have already modernised their legacy systems using AI.
JPMorgan’s COIN
JPMorgan Chase developed an AI-powered platform called COIN (Contract Intelligence) to automate the review of commercial loan agreements, which previously would take approximately 360,000 hours of legal and compliance work annually. COIN uses machine learning to interpret complex contracts in seconds, reducing human error and freeing up legal staff for more strategic responsibilities.
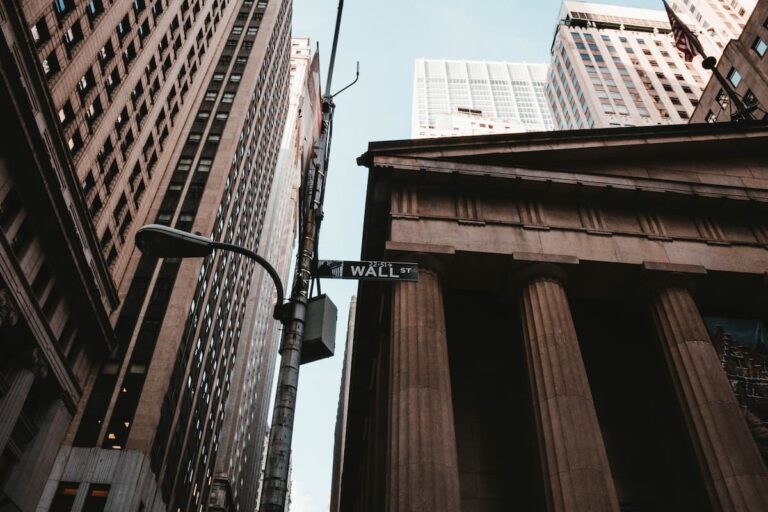
Beyond COIN, JPMorgan has invested in a team of over 9,000 experts to automate various aspects of its operations, including regulatory compliance and fraud detection. The initiative reflects the bank’s broader strategy to modernise its legacy systems and enhance efficiency through AI and automation.
New age of the NHS
The NHS is piloting AI-driven triage and navigation tools to replace outdated, manual processes that delay care and overwhelm staff. At the core of this initiative is a shift from fragmented, reactive systems to integrated, data-informed workflows that guide patients to the right services with minimal staff intervention.
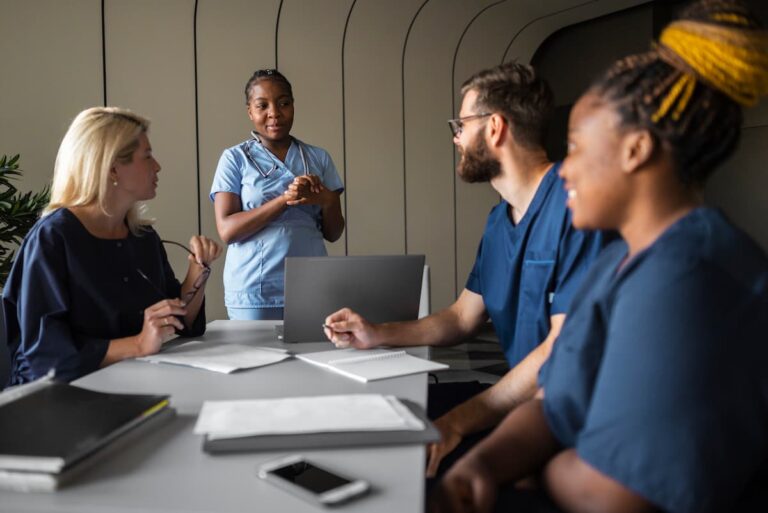
Smart Triage, one of the tested tools, allows up to 91% of appointments to be scheduled without human involvement. Wait times dropped from 11 to 3 days, and repeat appointments fell by 70%. These results show how AI can restructure front-door access to care by automating data collection, triage decisions, and referral routing.
Estimated annual savings of £340 million reflect both reduced administrative burden and more efficient use of clinical resources.
From legacy to quantum computing: Lufthansa’s story
Lufthansa is improving gate allocation with AI and quantum computing. Traditional methods, often manual and inefficient, are replaced by machine learning and quantum algorithms to optimise gate assignments. Shift aims to reduce aircraft taxi times, cut fuel use, and improve passenger transit times.
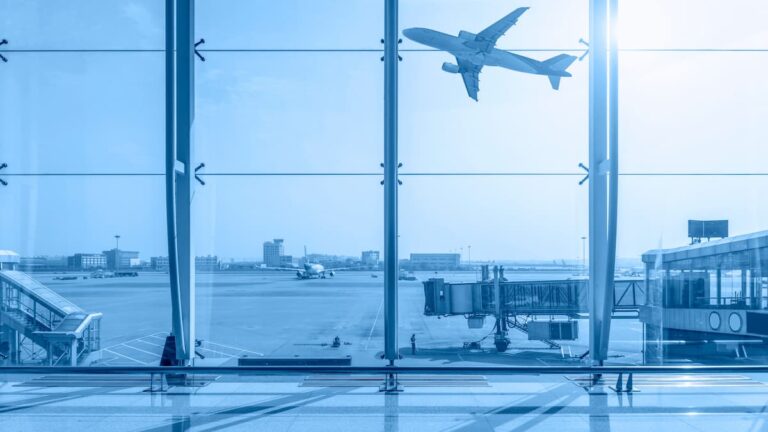
The AI system slashed gate assignment time from 4 hours to 10 minutes, saving 1.4 million gallons of jet fuel annually. Lufthansa’s quantum computing tests could reduce passenger transit times by nearly 50%.
To wrap up
Legacy systems are the backbone of a lot of companies. Usually, they can’t just be replaced, and the usual modernisation efforts might also not be an option. However, with AI, it all changes: it brings legacy systems up to speed, breathing a second life into the systems that weren’t supposed to handle modern pressure.
How Altamira can help
Whether you’re looking to modernise your legacy systems with AI, assess your current tech stack, or prototype your new ideas, our zero headaches approach guarantees to bring results, stress-free.
Tangible value: decade-long AI expertise and the business-first approach push technology to new heights.
Fast results: see the first clickable prototypes in days, not weeks.
Stay in the loop: know everything about your project through weekly meetings.
Get in touch to start building your AI-powered tools today.
FAQ
It’s about embedding AI capabilities into your existing systems, products, or workflows so they can do more—automatically. That might mean smarter search results, personalized recommendations, automated decision-making, or natural language interaction. It’s not just adding a model; it’s making sure AI fits into your tech stack and adds real value without breaking things.
Start by figuring out what problem you’re trying to solve. Then choose the right kind of model—prebuilt APIs, custom machine learning models, or even basic rule-based systems. Once you’ve got the right model, you need to connect it to your product or platform via APIs or SDKs, ensure it can access the right data, and build out logic to trigger its use. After that, you’ll want to test thoroughly and monitor how it performs in the real world.
First, be clear about what you want the AI to do. Do you want to classify emails? Generate text? Recommend content? Once that’s nailed down, look into tools that match your needs. For many use cases, you don’t need to train anything—you can use existing models through APIs (like OpenAI, Hugging Face, or Google). Add them into your app the way you’d add any external service, and be ready to tweak how they’re used based on user feedback.
AI integrators act as the bridge between machine learning models and working software. They make sure the AI fits with your systems, runs efficiently, and delivers what it’s supposed to. This often means choosing the right model, adapting it to your data and business logic, and embedding it into your architecture in a way that’s scalable and maintainable. It’s more engineering than magic—and it’s what turns an idea into something useful.